The Limitations of AI: Google DeepMind’s Discovery Challenges AGI Hype
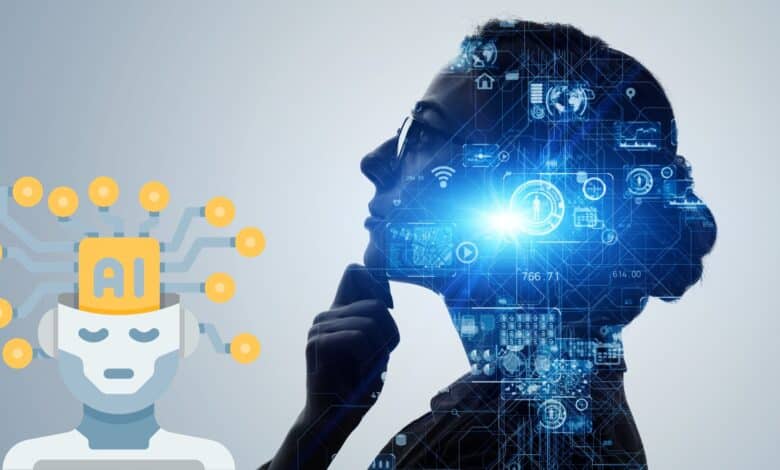
The limitations of AI, as highlighted in the recent research findings, underscore the challenges in achieving artificial general intelligence and emphasize the importance of realistic expectations in the field.
In a recent paper authored by Google DeepMind researchers Steve Yadlowsky, Lyric Doshi, and Nilesh Tripuraneni, a critical revelation about the current state of artificial intelligence (AI) models has surfaced. The focus of their study is on OpenAI’s GPT-2, a prominent transformer model that has been at the forefront of AI research. This not-yet-peer-reviewed paper sheds light on the challenges AI faces in generating outputs beyond its training data, raising questions about the widespread anticipation of achieving artificial general intelligence (AGI).
The Promise of Transformer Models
Transformer models, such as OpenAI’s GPT-2, have been hailed as the potential pathway to AGI. These models, conceived in a 2017 paper titled “Attention Is All You Need,” aim to mimic human-like intuitive thinking. The promise is immense—a machine capable of extrapolating and performing tasks beyond its training data would mark a significant leap in AI capabilities.
The Study’s Findings
However, the DeepMind researchers present a sobering reality. The paper illustrates that when faced with tasks outside the domain of their pre-training data, transformer models, including GPT-2, exhibit various failure modes and a degradation of generalization, even for seemingly simple extrapolation tasks. In simpler terms, if a transformer model lacks training on specific data, it struggles to perform tasks related to that data, regardless of their simplicity.
Implications for AI Enthusiasts
Despite the colossal training datasets used to build GPT-2, the findings suggest that current AI models are only proficient in areas they have been thoroughly trained on. The analogy of a well-educated child limited to the knowledge imparted in expensive preschools emphasizes that AI, like the child, relies heavily on the expertise of humans who contribute to its training data.
Challenges to AGI Aspirations
The study challenges the prevailing hype surrounding AGI. While CEOs like OpenAI’s Sam Altman and Microsoft’s Satya Nadella express plans to “build AGI together,” the research suggests a reality check. The paper underscores that the current approach to AI, represented by transformer models, falls short when confronted with tasks beyond their training scope.
Caution Amidst Hype
Since the release of ChatGPT, which is based on the GPT framework, pragmatic voices have urged a more tempered approach to AI expectations. The study aligns with these warnings, cautioning against presumptions of AGI capabilities and emphasizing the need for a realistic understanding of AI limitations.
Differing Perspectives in the AI Community
The divergence of opinions within the AI community is evident, with some researchers believing in the imminent achievement of AGI capabilities. However, the research by DeepMind employees challenges these optimistic views, suggesting that current transformer models may not possess the flexibility and adaptability required for true general intelligence.
Related articles: 10 Steps to Adopting Artificial Intelligence in Your Business
How To Make AI Work In Your Organization
FAQ
Q: What is the main finding of the Google DeepMind researchers’ paper?
A: The main finding of the paper is that current AI models, specifically transformer models like OpenAI’s GPT-2, struggle to generate outputs for tasks outside the scope of their training data. This limitation raises concerns about the feasibility of achieving artificial general intelligence (AGI), where machines exhibit human-like intuitive thinking.
Q: What is a transformer model, and why are they considered crucial for AGI?
A: Transformer models, like OpenAI’s GPT-2, are a type of AI model designed to transform one type of input into a different type of output. They are considered crucial for AGI because they are theorized to enable machines to undergo intuitive “thinking” similar to humans. The ability to make connections beyond training data is seen as a key step towards achieving AGI.
Q: How does the paper illustrate the limitations of transformer models, particularly GPT-2?
A: The paper demonstrates that when faced with tasks beyond their pre-training data, transformer models exhibit various failure modes and a degradation of generalization. Even for relatively simple extrapolation tasks, these models struggle to perform effectively, highlighting their current limitations.
Q: What analogy is used to explain the relationship between AI models and their training data?
A: The analogy used is that of a well-educated child sent to expensive and highly-rated preschools. Just as the child’s knowledge is limited to what is taught in those schools, AI models, despite extensive training datasets, are proficient only in areas they have been specifically trained on.
Q: How do the research findings impact the widespread anticipation of AGI?
A: The findings challenge the prevailing hype surrounding AGI by suggesting that the current approach, represented by transformer models, may not be as adaptable and flexible as needed for true general intelligence. The paper emphasizes the importance of a realistic understanding of AI limitations and urges caution in AGI expectations.
Q: What differing perspectives exist within the AI community regarding the achievement of AGI?
A: While some researchers, including CEOs like Sam Altman and Satya Nadella, express optimism about building AGI in the near future, the paper suggests a divergence of opinions. The DeepMind researchers’ findings imply that achieving AGI may be more challenging than anticipated, as current transformer models face significant hurdles in extrapolating beyond their training data.
Q: What is the broader implication of the research findings for the future of AI development?
A: The research findings highlight the need for a balanced perspective on AI advancements. As the race towards AGI continues, it underscores the importance of addressing challenges related to adaptability and extrapolation beyond training data. The findings may influence the direction of future AI research and development, prompting a more nuanced and cautious approach.
Conclusion
DeepMind researchers’ findings bring attention to a critical aspect of AI limitations. The study suggests that, at least for now, AI’s impressive feats are confined to the knowledge it has been trained on. As the race toward AGI continues, these findings serve as a reminder to approach AI advancements with a balanced perspective, acknowledging both its capabilities and constraints. While the dream of achieving artificial general intelligence persists, it seems the path forward may require overcoming substantial hurdles in the realm of AI’s adaptability and extrapolation beyond training data.